Programmatic Generation of RPM-Style Puzzles (Symbolic Approaches)
1: General Context
First-Order Logic Constraint Generation (Wang & Su, 2015)
Formal Logic Rules: Wang & Su (IJCAI 2015) introduced a purely symbolic framework that models RPM puzzles with first-order logic. They generalized the classic rule types identified by Carpenter et al. (1990) into logical formulas, enabling representation of any Advanced RPM problem (Automatic Generation of Raven's Progressive Matrices) (RAVEN: A Dataset for Relational and Analogical Visual REasoNing). Each puzzle’s structure and rules are formalized as logical constraints (predicates over object attributes like shape, size, number, etc.). This approach explicitly defines well-formedness conditions to ensure each generated puzzle is unambiguous (i.e. has a unique correct answer).
Enumerating Self-Consistent Completions: The generator treats puzzle completion as a constraint-satisfaction problem. All possible “answer” candidates that satisfy different subsets of the puzzle’s rules are systematically enumerated, and then filtered to ensure uniqueness. In particular, the method enforces two key properties: (1) a Correctness condition – only one answer satisfies all the logical constraints, and (2) a Necessity condition – if any rule (constraint) is omitted, there should be multiple possible answers. In practice, the algorithm constructs the correct answer to satisfy the full constraint set, then generates distractor answers by satisfying every proper subset of the constraints (i.e. leaving out one rule at a time). This guarantees that every rule is required for disambiguation: the correct panel is the only one meeting all constraints, while each wrong option violates at least one rule but may fulfill the others. The result is an RPM generator that produces puzzles with a unique, logically deducible solution, mirroring the difficulty and diversity of human-designed RPMs.
Procedurally Generated Matrices (PGM, 2018)
Relation-Triplet Framework: Barrett et al. (DeepMind 2018) created the PGM benchmark by procedurally generating RPM-like puzzles using a fixed set of symbolic relations. Each puzzle is defined by 1–4 underlying relation triples ((r, o, a)), where r is a relation type, o an object class, and a an attribute (Measuring Abstract Reasoning in Neural Networks - Amélie Royer). For example, a triple (progression, lines, number) denotes a rule that the number of line segments in each panel follows a progression across the row. A library of about 29 possible triples was used (some relations don’t apply to certain attributes).
Supported relation types include arithmetic progressions, logical operations (XOR, OR, AND), and set unions, applied to attributes like shape type, size, number of objects, position, or color. Unused attributes in a puzzle are either held constant or randomized as visual distractors (noise).
Ensuring Unique Solutions: The PGM generator instantiates each chosen relation triple to fill the 3×3 matrix, then constructs a set of 8 answer choices such that only one panel satisfies all the relations simultaneously. Each incorrect option typically satisfies a subset of the relations but fails one key rule, making it a plausible distractor. This design parallels the “monotonicity” principle: the correct answer maximizes rule satisfaction, and any candidate missing a rule is invalid. By encoding abstract relations symbolically and controlling attribute values, PGM ensures that the solution is logically determined by the strongest combination of relations. The PGM dataset (1.2M puzzles) was released for research, providing a large-scale testbed for analogical reasoning (GitHub - google-deepmind/abstract-reasoning-matrices: Progressive matrices dataset, as described in: Measuring abstract reasoning in neural networks (Barrett, Hill, Santoro*, Morcos, Lillicrap), ICML2018). Its symbolic generation method guarantees that solving an PGM puzzle requires deciphering the intended relations rather than exploiting spurious visual cues.
RAVEN Dataset: Grammar-Based Generation (2019)
Attributed Grammar Approach: Zhang et al. (CVPR 2019) developed RAVEN, a generator that adds a hierarchical visual structure to RPM puzzles. They use an Attributed Stochastic Image Grammar (A-SIG) to represent each puzzle as a parse-tree of components (RAVEN: A Dataset for Relational and Analogical Visual REasoNing) (RAVEN: A Dataset for Relational and Analogical Visual REasoNing). This grammar encodes figure configuration (seven layout templates, such as a centered single object, 2×2 grid, 3×3 grid, inside-outside nesting, etc.), and within each panel it instantiates one or more geometric objects. For each object attribute (e.g. shape type, number of objects, size, color), a rule type is sampled from Carpenter’s taxonomy. RAVEN implements four major rule families – Constant, Progression, Arithmetic (e.g. XOR/AND), and Distribute Three (i.e. “fill all three with one missing” pattern) – with slight parameter variations for diversity. These rules are applied row-wise to the grammar’s terminals, producing the 8 context panels such that each row (and column) obeys the chosen attribute relations. Crucially, RAVEN separates structural layout from rule logic – the generative grammar ensures complex layouts (e.g. objects inside others, or in different positions) without breaking the underlying symbolic rules.
Monotonic Answer Generation: To guarantee a unique correct answer, RAVEN explicitly adopts the monotonicity constraint strategy from Wang & Su. After generating the complete 3×3 matrix (with the true solution panel), the algorithm creates distractors by altering the solution’s attributes one rule at a time. In practice, for each underlying rule, one attribute in the correct answer (the one governed by that rule) is modified so that this new panel violates that single rule while preserving the others. This yields a set of alternative answers where each wrong choice “misses” one relational constraint. As a result, only the original solution panel satisfies the full set of attribute rules, ensuring an unambiguous completion. RAVEN’s approach thus marries a rich visual grammar with symbolic rules and a rigorous answer set construction, producing puzzles that require interpreting both the structural layout and the symbolic variations. The RAVEN dataset (generated with this method) contains 70k RPM problems across the 7 figure configurations, with annotated ground-truth rules for each puzzle.
Impartial RAVEN and Other Extensions
Avoiding Biased Solutions: Subsequent benchmarks identified that the original RAVEN answer sets had unintended biases (allowing shortcuts for machine-solvers). Impartial RAVEN (I-RAVEN) addressed this by regenerating answer choices to remove any superficial cues. In I-RAVEN, each question’s 8 options are constructed so that all share similar feature distributions, except the correct one uniquely fits the logic (I-RAVEN Dataset | Papers With Code). This makes the puzzle truly impartial: a solver cannot rely on single-attribute oddities (like one option having an extra shape) but must discern the full relational pattern. In essence, I-RAVEN follows the same symbolic generation of puzzles as RAVEN but with a more rigorous false answer generation (often by applying all monotonic rule-omission cases, as in Wang & Su’s original method). Likewise, a “RAVEN-FAIR” variant was introduced with balanced answer set sampling to further ensure no answer stands out except by rule satisfaction. These improvements underscore the importance of enumerating all self-consistent partial models (each missing one rule) so that the correct answer is only self-consistent when all rules are considered.
Open-Source Tools and Implementations
Several resources are available for generating RPM-style puzzles using the above symbolic techniques:
Raven-gen Library: An open-source Python toolkit that reimplements RAVEN’s generation algorithm (raven-gen 0.3.2 on PyPI - Libraries.io - security & maintenance data for open source software). It provides a high-level API to create RPM matrices with custom rule sets and figure configurations. For example, users can specify a list of attribute-rule pairs (e.g. number of objects follows Progression, shape type follows Distribute-Three, etc.) and generate new puzzles on the fly (raven-gen 0.3.2 on PyPI - Libraries.io - security & maintenance data for open source software) (raven-gen 0.3.2 on PyPI - Libraries.io - security & maintenance data for open source software). Raven-gen produces the puzzle images and even enumerates a set of wrong alternatives via the same one-rule-variation principle, ensuring the solution’s uniqueness. This tool is directly based on the RAVEN paper’s code and supports all 7 RAVEN configurations and rule types.
PGM Dataset Release: The Procedurally Generated Matrices data by DeepMind is publicly available for research. While the original code was not fully open-sourced, the released dataset (over 1 million generated puzzles with labels for underlying triples) serves as a resource and reference implementation of the triple-based generation approach. Researchers can inspect these examples or use the provided TensorFlow dataset loader to understand PGM’s construction. The generation process (defining relation triples and sampling distractors) can be reimplemented following the descriptions in Barrett et al.’s paper.
Other Implementations: The first-order logic approach by Wang & Su was demonstrated in their 2015 study, and although their code isn’t publicly released, the methodology could be implemented with a logic programming or constraint-solving library. For instance, one could encode RPM rules in an SMT or Prolog solver to systematically generate matrices and then use a brute-force or optimized search (per the monotonicity proposition) to construct answer sets. Additionally, the literature includes open-source solvers for RPM (e.g. neuro-symbolic models, which often include re-generated puzzles or rule representations that can inspire a custom generator.
2: Human Solvers Identify Unintended Patterns
In my opinion, any RPM generation algorithm is susceptible to a basic flaw that arises from translating the underlying symbolic model into visuals: the generated visuals may suggest alternative valid solutions that the algorithm cannot see or reason about.
There is necessarily a mismatch between the symbolic logic underlying puzzle generation and the visual representation interpreted by human cognition. Symbolic algorithms generate puzzles by explicitly encoding a finite set of logical constraints—attributes, relationships, and transformations—into visual form. These constraints define a specific, intended solution. However, once these symbolic rules are instantiated visually, the puzzle representation often unintentionally encodes additional or ambiguous structural regularities that the algorithm does not explicitly model or detect.
From a symbolic standpoint, the generator strictly applies known rules (e.g., arithmetic progression of shapes or object placement logic). But visually, humans interpret these puzzles through pattern recognition heuristics that are inherently broader. They may identify emergent, unintended patterns involving symmetry, proportionality, repetition, spatial arrangements, or perceptual grouping—relationships the original generator neither anticipated nor explicitly tested.
As a result, a puzzle that the algorithm believes has a single valid solution may inadvertently admit multiple solutions, each coherent according to distinct self-consistent interpretations formed by the solver. These alternate solutions appear equally valid from the solver's perspective because they respect plausible visual symmetries or patterns that were never encoded symbolically in the generator's rules.
Implicit visual structure: The visual domain inherently supports richer relationships than symbolic encodings explicitly track. Spatial or perceptual relations (e.g., symmetry, alignment, proximity) naturally emerge as "accidental regularities."
Under-specification of symbolic constraints: Symbolic rules often incompletely specify visual configurations. Attributes considered "irrelevant" or randomly varied by the algorithm (like position or orientation) may inadvertently encode additional meaningful relationships.
Human cognitive flexibility: Human solvers readily infer multiple simultaneous, orthogonal hypotheses, even if these hypotheses span distinct conceptual models. Humans implicitly test each plausible model against visual evidence, selecting whichever interpretation yields coherence—even if the generator did not explicitly model or even recognize such an interpretation.
There are two obvious strategies to combat this:
A: severely restrict the visual output language to a "safe" subset, which is a hard problem in of itself.
B: algorithms beyond generating merely symbolics. They must additionally incorporate visual structural analyses capable of enumerating unintended patterns—effectively adopting a form of visual "proof checking" or "pattern enumeration." Such a mechanism would:
- Enumerate visual constraints explicitly, going beyond the original symbolic intent.
- Validate uniqueness visually by systematically testing for additional unintended, plausible regularities.
- Adjust the puzzle iteratively until all alternative plausible solutions (other than the intended one) become invalid, ensuring that visual encoding aligns exactly with symbolic intentions.
Is It Algorithmically Tractable to Restrict the Output Language to a "Safe" Ruleset?
We have demands and requirements!
Controlled combinatorial complexity:
A carefully curated rule set significantly reduces accidental visual regularities, making it feasible to enumerate or systematically validate all resulting visual configurations.Explicit enumeration & verification:
Because each "safe" rule can be thoroughly vetted beforehand, exhaustive testing becomes feasible. This allows pre-verifying all possible visual outcomes for hidden emergent properties.Tractable combinatorial explosion:
By limiting complexity and number of allowed interactions (e.g., avoid nested hierarchies, overlapping symmetries, or spatial regularities), combinational enumeration remains manageable.
Naive Methods
Define a constrained visual grammar:
- Clearly specified object relations: arithmetic progressions, rotations, translations, color shifts.
- Avoid ambiguous visual constructs: nested objects, overlapping, complex symmetries, or perceptually close alignments.
Formalize visual constraints symbolically:
- Employ constraint logic programming or SMT solvers to explicitly model spatial and perceptual constraints.
- Enforce constraints that explicitly forbid ambiguous or emergent visual properties.
Pre-validate and filter:
- Precompute or enumerate symbolic-visual correspondences exhaustively within this constrained grammar.
- Filter out configurations that fail uniqueness criteria at design-time.
I maintain the conjecture that any expansion of a "safe" ruleset carries an exponential increase in factors that would need to be considered by a generation algorithm. The choice here may well be between a basic ruleset that is too simple for both psychological testing or entertainment/gameplay, or one that is rich enough to be interesting but difficult-to-impossible to prove safe.
There is nothing worse in this context than a test or a game confidently asserting that there is only one valid solution to a problem when in reality there are more.
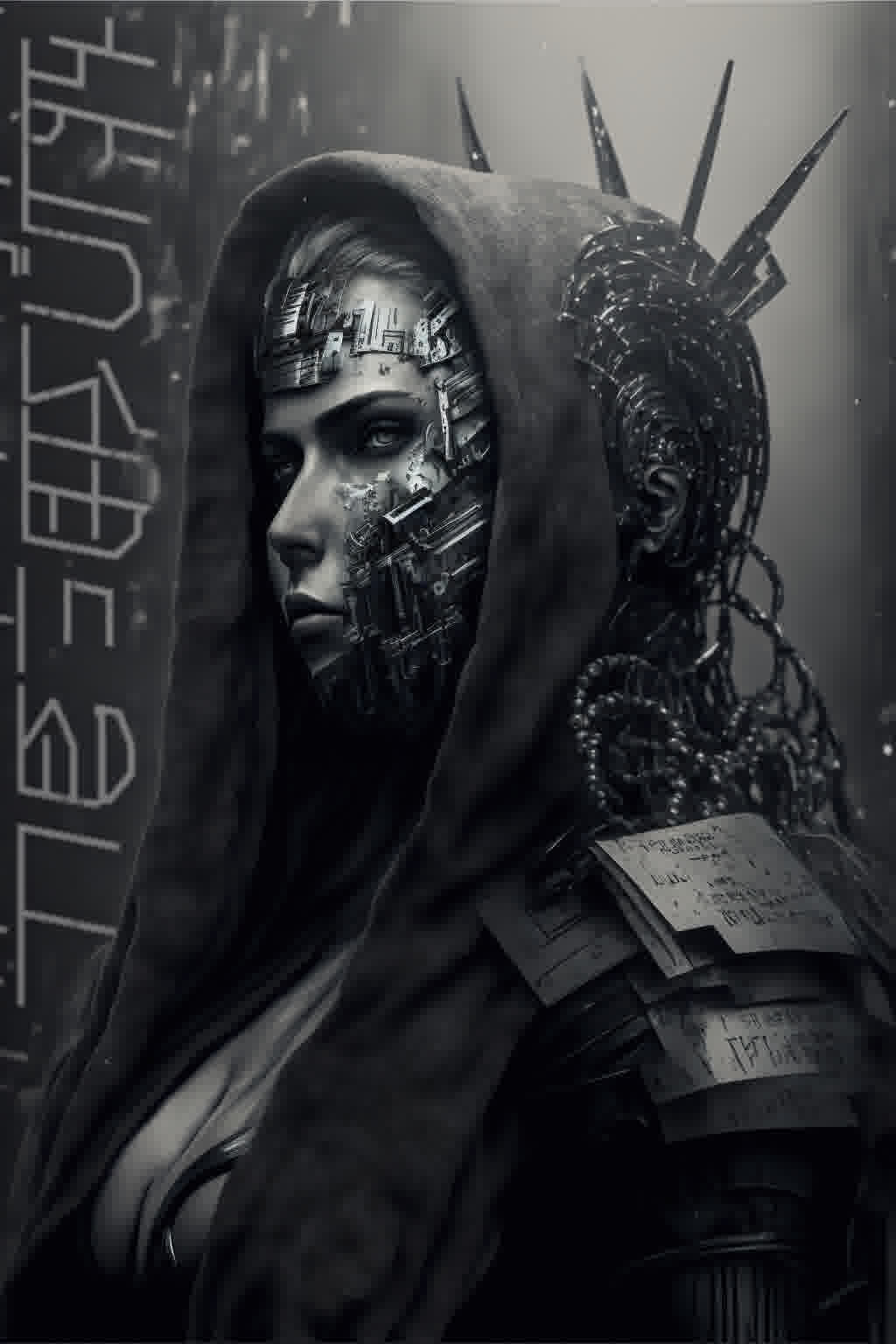